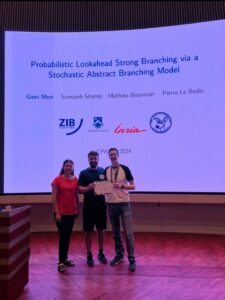
Gioni Mexi is a PhD student in the SynLab of the Research Campus MODAL, where he works on advancing the state of the art in mixed-integer linear programming. The paper titled “Probabilistic Lookahead Strong Branching via a Stochastic Abstract Branching Model” proposes a new strong branching method that is based on an abstract stochastic tree model where the dual gains of all variables follow a given probability distribution. This model allows to relate expected dual gains to tree sizes. This leads to a new stopping criterion for strong branching, which dynamically continues or interrupts strong branching. The new algorithm significantly improves both memory consumption and runtime. The work results from an international collaboration fostered by the MODAL workshop and guest programs.
The photo shows, from left to right, Bistra Dilkina, University of Southern California, USA, the chair of the CPAIOR 2024 program committee, Gioni Mexi, and Pierre Le Bodic, Monash University, Melbourne, Australia.
Congratulations!